Math in Science
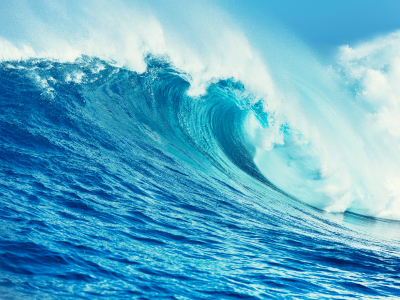
Equations
Statistics
Trigonometric Functions
Sign in or register
For an ad-free experience and access the Visionlearning Classroom, sign in or register.
For an ad-free experience and access the Visionlearning Classroom, sign in or register.